AI (Artificial Intelligence) is MACHINE USEFULNESS
Author(s)
Datta, Shoumen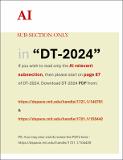
DownloadMachine Usefulness (206.5Kb)
Additional downloads
Terms of use
Metadata
Show full item recordAbstract
MACHINE USEFULNESS - according to Daron Acemoglu (2024 Nobel Prize) is more appropriate instead of the misnomers AI and ML. Hence, AI in need of an explanation as to why it is actually incorrect. But, if used with human supervision, AI tools, e.g., ANN/ML with humans-in-the-loop (ML-HIL) can be made very useful when dealing with very high volume of data and data analytics. Therefore, machines are useful but not in the way it is conceived in the AI/ML jargon.
Description
Harvard Law Professor Paul Freund, a constitutional scholar, asserted that the Supreme Court “should never be influenced by the weather of the day but inevitably will be influenced by the climate of the era.” But, unlike law (interpretation “will be influenced by the climate of the era”), in science neither “weather of the day” nor “climate of the era” may influence evidence. So-called “deep learning” (convolutional neural network) was inspired by the work of David Hubel and Torsten Weisel (in 1962) while exploring the neural architecture of the cat’s visual cortex in the 20th century. In the 21st century, Halicin sets the “gold standard” for exceptionally brilliant use of un-intelligent and dumb tools of ANN/ML to extract suggestive analytics based on human-designed training and learning. The success of ANN/ML in high volume, human-in-the-loop data handling, in the case of Halicin is a clarion call to dispense with the hubris of AI (and AI politics). However, it is impossible not to take note that AI politics is not a domain where evidence plays any role. AI has become a domain for pompous puffery.
The evidence from the cat's visual cortex (in the 20th century) in combination with the repurposed antibiotic Halicin and the pitfalls of ML in genomics/proteomics (in the 21st century) may be sufficient to suggest that AI shenanigans are unnecessary.
Date issued
2024-07-03Keywords
Machine Usefulness, Daron Acemoglu, AI, ML, DL, RL, ANN, CNN, RNN, MPNN, DNN, Halicin, Digital Twins, Digital Cousins, Data, Data Analytics, SGD, Stochastic Gradient Descent, Back Propagation Algorithm, GARCH, Independent Variable, Halicin, genomics, proteomics, visual cortex, David Hubel, Torsten Wiesel, Clive Granger, causality
Collections
The following license files are associated with this item: