EuroPED-NN: Uncertainty aware surrogate model
Author(s)
Panera Alarez, A.; Ho, Aaron; Järvinen, A.; Saarelma, S.; Wiesen, S.; JET contributors; ASDEX Upgrade Team; ... Show more Show less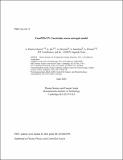
Download24ja071_full.pdf (4.307Mb)
Metadata
Show full item recordAbstract
This work successfully generates an uncertainty-aware surrogate model of the EuroPED plasma pedestal model using the Bayesian neural network with noise contrastive prior (BNN-NCP) technique. This model is trained using data from the JET-ILW pedestal database and subsequent model evaluations, conforming to EuroPED-NN. The BNN-NCP technique has been proven to be a suitable method for generating uncertainty-aware surrogate models. It matches the output results of a regular neural network while providing confidence estimates for predictions as uncertainties. Additionally, it highlights out-of-distribution (OOD) regions using surrogate model uncertainties. This provides critical insights into model robustness and reliability. EuroPED-NN has been physically validated, first, analyzing electron density ne(ψ_pol = 0.94) with respect to increasing plasma current, Ip, and second, validating the Δ−β_p,ped relation associated with the EuroPED model. This affirms the robustness of the underlying physics learned by the surrogate model. On top of that, the method was used to develop a EuroPED-like model fed with experimental data, i.e. an uncertainty aware experimental model, which is functional in JET database. Both models have been also tested in ~50 AUG shots.
Description
Submitted for publication in Plasma Physics and Controlled Fusion
Date issued
2024-06Department
Massachusetts Institute of Technology. Plasma Science and Fusion CenterJournal
Plasma Physics and Controlled Fusion
Publisher
IOP
Other identifiers
24ja071